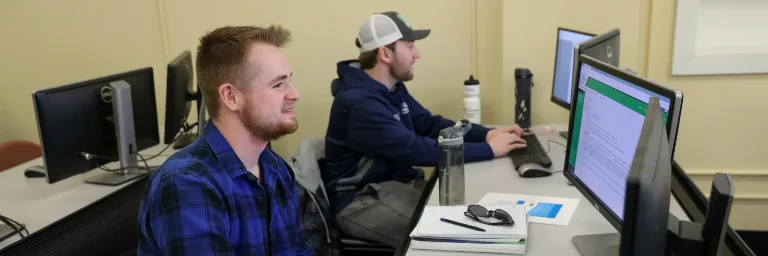
Data Science vs. Data Analytics: What’s the Difference?
The origins of data science are deeply rooted in the convergence of computer science and statistics.
Request Information
In the early days of computing, statisticians quickly recognized the profound potential of computers to enhance data analysis. Then, as data science continued to evolve, a complementary discipline began to take shape—data analytics.
While both fields deal with data, they exhibit distinctive characteristics and cater to different objectives, ultimately leading to divergent career paths. Continue reading to explore the key distinctions between data science vs. data analytics and determine which path aligns with your career aspirations.
What is Data Science?
Data science is an interdisciplinary field that focuses on extracting knowledge and insights from structured and unstructured data. Using various techniques, from statistics, machine learning and data mining, data scientists collect, clean and analyze data to make predictions and inform strategic decision-making. The process typically includes data collection, rigorous data cleaning, deep analysis and the interpretation of the results.
This field applies to virtually every industry, including finance, healthcare and technology, showcasing its wide-ranging utility and importance in solving complex problems.
What is Data Analytics?
Data analytics is the systematic examination of datasets to uncover valuable insights and informed conclusions, crucial for strategic decision-making in organizations.
The discipline includes several distinct approaches. Descriptive analytics summarizes past data to clarify what has occurred, setting the stage for diagnostic analytics, which probes into the causes behind these events. Building on this understanding, predictive analytics uses historical data to anticipate future outcomes. Finally, prescriptive analytics offers targeted strategies based on these forecasts, helping organizations achieve optimal results.
This progression from understanding what has happened to shaping future outcomes is vital for enhancing performance and sparking innovation across various industries.
Education Requirements
Pursuing a data science or analytics career requires a solid educational foundation in relevant fields. Each area has its specific educational paths that help aspirants acquire the necessary skills and knowledge.
Data Science Education
The journey into data science typically begins with an undergraduate degree in Data Science, Computer Science, statistics, Mathematics or a related field. These programs lay the groundwork by equipping students with essential mathematical and computational skills crucial for handling and analyzing complex data sets.
To deepen their knowledge and specialize further, many individuals pursue graduate degrees, such as a master's degree in Data Science or related fields. These programs often focus on advanced topics like machine learning, big data technologies, artificial intelligence and sophisticated statistical methods.
Those looking to push the boundaries of data science may even opt for Ph.D. programs, which are research-focused and allow for specialization in cutting-edge areas such as deep learning, natural language processing or cognitive computing.
Data Analytics Education
Aspiring data analysts often start with undergraduate degrees in Business Analytics, Information Systems, Statistics or similar fields. These degrees provide a solid foundation for understanding how to collect, process and analyze data as well as apply it in a business context to solve problems and support decision-making.
Advancing in data analytics might involve pursuing a master's degree in Data Analytics, Business Intelligence or Applied Statistics. These programs typically focus on more strategic aspects of data analytics, including advanced statistical methods, predictive analytics and the use of analytics in business strategy. Post-graduate certifications in tools and technologies such as SQL, Tableau, SAS or Power BI are also highly valued in the industry.
Skill Set Requirements
Both data science and data analytics professionals require a specific set of skills; however, some of these skills overlap, reflecting the integrated nature of these disciplines.
Data Science Skills
Data scientists are expected to possess a wide array of skills, each critical for various aspects of their role:
- Programming languages: Python and R for coding and data manipulation
- Machine learning: Application of algorithms and models for complex problem-solving
- Data visualization: Creation of visual representations to clarify and highlight data trends
- Domain expertise: Deep industry knowledge to effectively apply data insights
- Data analysis: Interpretation of complex datasets to extract actionable information
- Big data tools: Proficiency with platforms like Hadoop and Spark for handling large data volumes
- Deep learning frameworks: Familiarity with sophisticated models for advanced data analysis
- Statistical analysis: Application of statistical methods for meaningful data interpretation
Data Analytics Skills
Data analysts require several core competencies to effectively handle data:
- Data manipulation: Proficiency in cleaning, sorting, and organizing data
- Database management: Skilled in using SQL for efficient data retrieval and management
- Data visualization: Ability to create clear and impactful visual representations of data
- Data interpretation: Competence in analyzing large datasets to extract meaningful insights
- Analytical thinking: Strong critical thinking skills to evaluate data and assess implications
- Communication: Capability to translate technical data findings into understandable and actionable business insights
- Statistical analysis: Solid understanding of statistical methods to conduct accurate data evaluations
Career Opportunities
The fields of data science and data analytics offer varied career opportunities, some overlapping while others are unique to each field.
Data Science Careers
Data science offers a range of career paths, including:
- Data scientist
- Machine learning engineer
- Artificial intelligence specialist
- Deep learning engineer
- Big data engineer
- Algorithm developer
These roles typically focus on the development of new algorithms, predictive models and systems for data analysis and interpretation, pushing the boundaries of what organizations can achieve with data.
Data Analytics Careers
In data analytics, the roles are often more directly linked to business applications and include:
- Data analyst
- Business intelligence analyst
- Data engineer
- Marketing analyst
- Financial analyst
These professionals are crucial in analyzing data to inform business strategies, improve operational efficiency and enhance market understanding across various industries, such as finance, marketing and healthcare.
Salary and Job Outlook
The growing emphasis on data has propelled the fields of data science and data analytics into the spotlight, predicting a promising future for both domains. Not only are these roles expected to remain in high demand, but they also offer attractive salaries and substantial career growth opportunities.
Data Dcience Jobs
The role of a data scientist is not only intellectually rewarding but also financially lucrative, with an average salary of approximately $108,020 per year. The job market for data scientists is robust, boasting about 168,900 professionals engaged in this dynamic field. Looking ahead, the outlook for data science is exceptionally bright, with employment projected to grow at a rate of 35% from 2022 to 2032, a pace much faster than the average for all occupations.
Data Analytics Jobs
Similarly, data analytics professionals enjoy competitive salaries, with an average annual income of around $77,445. The salary can vary depending on factors such as experience, location and the specific industry in which one works.
The job market for data analysts is also experiencing a significant upswing and is projected to grow by 25% by 2030. This expansion is a testament to the growing reliance on data analytics to inform and shape business decisions.
Challenges and Future Trends
Professionals in data science and analytics face numerous challenges, including maintaining data quality, managing privacy concerns and overcoming scalability issues. As data volumes continue to grow, keeping data accurate and clean becomes even more challenging.
Privacy concerns are also significant as both fields handle sensitive information, requiring compliance with increasingly stringent regulations. Scalability is another hurdle, as systems must be designed to handle large-scale data analysis.
Several trends and technologies are shaping the future of data science and data analytics. For instance, the advancement of AI and machine learning is expanding the capabilities and efficiency of data analysis. Big data analytics is also becoming more sophisticated, providing deeper insights and predictive capabilities. Additionally, there is a growing focus on the ethical aspects of data usage, pushing for transparency and fairness in data-driven decision-making.
Data Science vs. Data Analytics: Which One Should You Choose?
Choosing between a career in data science and analytics depends largely on your interests, strengths and aspirations. Data science might be the right path if you are drawn to complex mathematical models, deep statistical analysis and building algorithms.
On the other hand, if you are interested in using data to solve business problems, improve operational efficiencies and support decision-making, then a career in data analytics could be more suitable.
To make an informed decision, assess your skills in programming, mathematical analysis and interest in business applications. Reflect on whether you thrive in abstract mathematical concepts or prefer the tangible impact of data-driven solutions in business contexts. Also, consider your long-term career aspirations and how each path aligns with your goals.
Keep in mind that the fields of data science and data analytics are not mutually exclusive, and there is often overlap between the two. Ultimately, choose the path that resonates most with your passion, strengths and professional aspirations.
Conclusion
All in all, while both data science and data analytics revolve around the use of data, they differ significantly in scope and application. But, regardless of which path you choose, each offers a wealth of opportunities and the potential for a rewarding career in a field that is only expected to grow in relevance and demand.
Remember, in a world increasingly driven by data, your ability to analyze and interpret this vital resource can set you apart and propel you to success. Let UND help you unlock the full potential of data in your professional journey. Explore our cutting-edge programs to gain the expertise and skills necessary to thrive in these fields.
FAQs
Data science often utilizes tools such as Python, R, TensorFlow and Apache Hadoop, focusing on machine learning, deep learning and big data technologies. In contrast, data analytics frequently employs SQL, Tableau, Power BI and Excel, emphasizing data manipulation, visualization and reporting.
Data science is particularly prevalent in industries like technology, healthcare and finance, where predictive modeling and deep learning are crucial. Data analytics is more widespread in business sectors such as retail, e-commerce and marketing, where operational decisions and consumer behavior analysis are key.
Data science and analytics teams work closely with marketing, finance and operations to provide insights that inform strategies and improve efficiency. They help these departments by analyzing trends, forecasting outcomes and optimizing processes based on data-driven evidence.